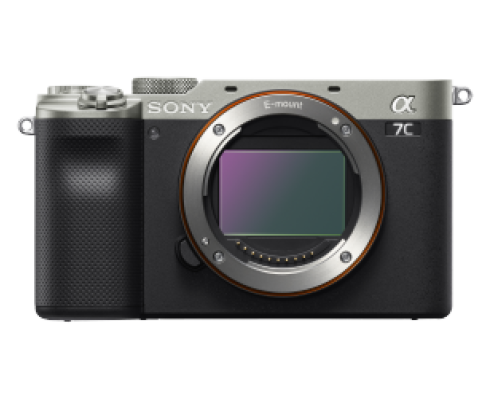
Sony Alpha camera supported by Sony SDK (Software Development Kit)
Sony Alpha camera supported by Sony SDK (Software Development Kit)
Digital Video Camera using various interfaces (USB, Gigabit Ethernet, Camera link)
By Arnaud Destruels, MV Product Marketing Manager, Sony Image Sensing Solution Europe
Efficiency is a key factor in driving the deployment of machine-vision systems. The systems improve productivity and profitability through the reduction of waste. Achieving efficiency in machine vision is a multi-faceted challenge. The efficient deployment of machine vision in many industrial scenarios involves more than simply designing in a high-resolution image sensor. Numerous system-level issues determine how effective the imaging solution will be in its environment, from mechanical fixtures through to the system’s ability to deliver consistent results at high speed.
A major driving force in industrial machine vision is the pressure to reduce the amount of time required to recognise what is needed to correctly deal with a product or subsystem as it passes through the manufacturing process. It may be damaged, unfinished or be fitted with the wrong options. The quicker that the product can be pulled from the mainstream flow and passed to a station for remedial action or rejected the more efficient the overall process.
To ensure accurate capture and ensure that all out-of-tolerance products are flagged correctly, consistency needs to be maintained. At high speeds, there is a risk that a defective product will be incorrectly flagged as healthy because an image of it cannot be captured correctly or that healthy products are flagged falsely because a mistake or delays in triggering image capture causes the recognition software to misread the image as that of a defective item. If there is no way to ensure reliable high-speed operation, the entire production line has to be slowed down to accommodate the inspection systems.
A better alternative is to examine the system architecture and implement changes that lead to greater accuracy and repeatability. Both spatial and temporal accuracy are vital to guaranteeing the system performance needed for high-throughput manufacturing. The architecture needs to deal with issues such as false triggering and data link congestion.
False triggering of an image sensor can be caused by electrical noise in the industrial environment. This can lead to images that, because the objects in them are improperly registered, can lead the software to conclude that the nearest product on the line has been damaged. At best, the image increases network congestion that can delay other important data that needs to pass across the network. A trigger-range function deals with the problem of false triggering by only starting a capture if the trigger signal lasts longer than a pre-set period. Any shorter trigger signals can be disregarded as noise.
Real-time machine vision has often been achieved through the use of point-to-point links between cameras and image-processing subsystems. But this limits the connection topology and makes it difficult to share images between different processing engines, which may be important in situations where comparisons are important or parallel processing is required for high throughput. A network-based topology provides much greater freedom but it introduces the problem of congestion when multiple cameras are trying to send images at the same time.
Ethernet provides the opportunity to build practically any form of logical topology from stars to highly interconnected meshes on its core hub-and-spoke physical organisation. Ethernet allows spans of up to 100m between network nodes and hubs over standard, low-cost twisted-pair cabling with high noise resilience. Where greater electrical noise immunity is required or for greater reach from the hub to the network node fibre connections can be used.
As a standard supported by most computer hardware, it is an excellent choice for interoperability and has demonstrated time and again its ability to evolve with requirements, such as the introduction of Gigabit Ethernet, pushing data rates to 1Gb/s. This high-speed version of Ethernet has, in turn, been incorporated into the GigE Vision group of standards that promote a collection of technologies that support high-throughput imaging.
As well as Gigabit Ethernet, a key protocol within GigE Vision is the IEEE 1588 Precision Time Protocol. This enhances accuracy in production by ensuring that every system on the network is synchronised to a common high-accuracy clock signal that is resistant to network delays. The protocol helps ensure that image capture is precisely aligned with processing. IEEE 1588 allows a precise time stamp to be added to each image so that the system can link images and results to a specific object on the production line so that it can be marked electronically for removal or for further manipulation by a robot downstream. In addition, it provides a high-precision mechanism for triggering image capture by software across multiple cameras without demanding separate point-to-point hardware connections from a controller to the individual sensors.
GigE Vision further promotes interoperability in machine vision by incorporating the Generic Interface for Cameras (GeniCam) standard developed by the European Machine Vision Association (EMVA) for software development. By adopting open software standards, camera and other subsystem manufacturers can ensure integrators and end users are provided with a straightforward migration path towards improved features, resolution and system capability.
GenICam provides supports for five basic functions that help speed up machine-vision configuration. Standard APIs are used to configure the camera to make it possible to support a range of camera features such as frame size, acquisition speed and pixel format and capture images from them. Further APIs provide support for sending extra data with an image, such as acquisition parameters, time stamps and areas of interest in the frame. The APIs also provide a means to set up and handle events, such as capture triggers.
The high-speed data transfer of Gigabit Ethernet coupled with the temporal accuracy offered by IEEE1588 supports temporal consistency in machine vision. Spatial accuracy is just as important in supporting high throughput and low takt times. A high degree of image repeatability is important as this reduces the probability of misrecognition and cuts the computational burden on image processing systems. This is because the spatial repeatability eliminates the need to perform translation and rotation corrections on captured images that might be needed otherwise.
The need for spatial consistency and accuracy translates into requirements for the mechanical design of image sensors. This is why the GS CMOS cameras made by Sony use a highly regular cube-shape format that is designed deliberately to be easy to fix mechanically. Furthermore the camera modules are made with extremely fine tolerances on their mounting points to ensure high spatial consistency in assembled subsystems.
By taking system-level considerations into account when designing and specifying machine-vision systems, the user can be sure that the results will support high-throughput operation and the low takt times that are now required in a wide variety of industrial scenarios. The support built into Sony’s XCG and XCL cameras ensures users and integrators have a straightforward means of making the right system-level decisions.
For more information see http://www.image-sensing-solutions.eu for more details.
For further editorial information, please contact:
Rob Ashwell, Publitek
+44 (0) 1225 470000 / rob.ashwell@publitek.com /
For further product and sales information, please contact:
Matthew Swinney, Senior Marketing Manager, Sony Image Sensing Solutions.
Tel. +44 (0) 1932 817494 / matthew.swinney@eu.sony.com